2020 Symposium Speakers
Keynote Speaker
Associate Professor, Department of Civil and Systems Engineering
Co-director, Center for Systems Science and Engineering
Affiliated Faculty, Infectious Disease Dynamics Group – Bloomberg School of Public Health
CSIRO Visiting Scientist
COVID Dashboard
Lauren Gardner, associate professor in the Department of Civil and Systems Engineering at Johns Hopkins Whiting School of Engineering, is the creator of the interactive web-based dashboard being used by public health authorities, researchers, and the general public around the globe to track the outbreak of the novel coronavirus. The dashboard, which debuted on January 22, became the authoritative source of global COVID-19 epidemiological data for public health policy makers and many major news outlets worldwide. Because of her expertise, Gardner was one of six Johns Hopkins experts who briefed congressional staff about the outbreak during a Capitol Hill event in early March 2020.
2020 Symposium Speakers
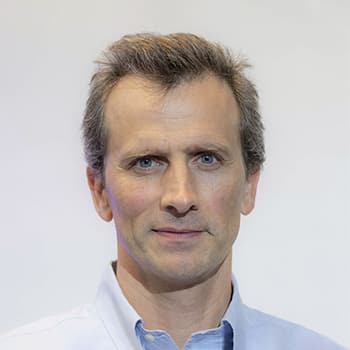
Research Faculty, INRIA – National Institute for Research in Digital Science and Technology,
Paris, France
Scientific Leader, SIERRA – Machine learning research laboratory
Many supervised learning methods are naturally cast as optimization problems. For prediction models which are linear in their parameters, this often leads to convex problems for which many guarantees exist. Models which are non-linear in their parameters such as neural networks lead to non-convex optimization problems for which guarantees are harder to obtain. In this talk, I will consider two-layer neural networks with homogeneous activation functions where the number of hidden neurons tends to infinity, and show how qualitative convergence guarantees may be derived. I will also highlight open problems related to the quantitative behavior of gradient descent for such models. (Joint work with Lénaïc Chizat)
Francis Bach is a researcher at Inria, leading since 2011 the machine learning team which is part of the Computer Science department at Ecole Normale Supérieure. He graduated from Ecole Polytechnique in 1997 and completed his Ph.D. in Computer Science at U.C. Berkeley in 2005, working with Professor Michael Jordan. He spent two years in the Mathematical Morphology group at Ecole des Mines de Paris, then he joined the computer vision project-team at Inria/Ecole Normale Supérieure from 2007 to 2010. Francis Bach is primarily interested in machine learning, and especially in sparse methods, kernel-based learning, large-scale optimization, computer vision and signal processing. He obtained in 2009 a Starting Grant and in 2016 a Consolidator Grant from the European Research Council, and received the Inria young researcher prize in 2012, the ICML test-of-time award in 2014, as well as the Lagrange prize in continuous optimization in 2018, and the Jean-Jacques Moreau prize in 2019. He was elected in 2020 at the French Academy of Sciences. In 2015, he was program co-chair of the International Conference in Machine learning (ICML), and general chair in 2018; he is now co-editor-in-chief of the Journal of Machine Learning Research.
Professor of Mechanical Engineering at Stanford University
Director, Institute for Computational and Mathematical Engineering
95% of today’s data has been created in the last three years! Object recognition, health monitoring, language translation are only three examples of how society is being transformed in the age of data. The exponential growth of interest in data is fueled by three elements
(1) the introductions of new, inexpensive sensors and diagnostic technology,
(2) the availability of high-quality, general-purpose and open source machine learning tools and
(3) the development of novel computer architectures tailored for handling data tasks.
How science and technology innovation will change in the age of the data is still somewhat unclear and the current impact is more subtle, partly because of existing practices based on well-established physical and mathematical principles. The talk will provide a historical perspective on the use of data in engineering simulations for validation, calibration and inference. This will be contrasted with new emerging trends in data science and specifically in merging mathematical models with data.
Many of the examples described emerge from the use of data science tools in simulations of turbulence.
Gianluca Iaccarino is Director of the Institute for Computational Mathematical Engineering and a professor in the Mechanical Engineering Department at Stanford University. He received his PhD in Italy from the Politecnico di Bari (Italy) and has worked for several years at the Center for Turbulence Research (NASA Ames & Stanford) before joining the faculty at Stanford in 2007.
Since 2014, he is the Director of the PSAAP Center at Stanford, funded by the US Department of
Energy focused on multi-physics simulations, uncertainty quantification and exascale computing . In 2010, he received the Presidential Early Career Award for Scientists and Engineers (PECASE) award, in the last couple of years, he has received best paper awards from AIAA, ASME and Turbo Expo Conferences.
Professor in Department of Computer Science and Department of Statistics
Senior Data Science Fellow, eScience Institute
Adjunct Professor in Department of Electrical Engineering, University of Washington
The area of offline reinforcement learning seeks to utilize offline (observational) data to guide the learning of (causal) sequential decision making strategies. It is becoming increasingly important to numerous areas of science, engineering, and technology. Here, the hope is that function approximation methods (to deal with the curse of dimensionality) coupled with offline reinforcement learning strategies can provide a means to help alleviate the excessive sample complexity burden in modern sequential decision making problems. However, the extent to which this broader approach can be effective is not well understood, where the literature largely consists of sufficient conditions.
This talk will focus on the basic question of what are necessary representational and distributional conditions that permit provable sample-efficient offline reinforcement learning? Perhaps surprisingly, our main result shows even if: i) we have realizability in that the true value function of _all_ target policies are linear in a given set of features and 2) our off-policy data has good coverage over all these features (in a precisely defined and strong sense), any algorithm information-theoretically still requires an exponential number of offline samples to non-trivially estimate the value of the _any_ target policy. Our results highlight that sample-efficient, offline RL is simply not possible unless significantly stronger conditions hold: such as either having extremely low distribution shift (where the offline data distribution is close to the distribution of the target) or far stronger representational conditions must hold (beyond realizability).
Sham Kakade is a professor in the Department of Computer Science and the Department of Statistics at the University of Washington and is also a senior principal researcher at Microsoft Research. His works is on the mathematical foundations of machine learning and AI. Sham’s thesis helped in laying the statistical foundations of reinforcement learning. With his collaborators, his additional contributions include: one of the first provably efficient policy search methods in reinforcement learning; developing the mathematical foundations for the widely used linear bandit models and the Gaussian process bandit models; the tensor and spectral methodologies for provable estimation of latent variable models; the first sharp analysis of the perturbed gradient descent algorithm, along with the design and analysis of numerous other convex and non-convex algorithms. He is the recipient of the ICML Test of Time Award, the IBM Pat Goldberg best paper award, and INFORMS Revenue Management and Pricing Prize. He has been program chair for COLT 2011.
Sham was an undergraduate at Caltech, where he studied physics and worked under the guidance of John Preskill in quantum computing. He then completed his Ph.D. Peter Dayan in computational neuroscience at the Gatsby Unit at University College London. He was a postdoc with Michael Kearns at the University of Pennsylvania. Sham has been a Principal Research Scientist at Microsoft Research, New England, an associate professor at the Department of Statistics, Wharton, UPenn, and an assistant professor at the Toyota Technological Institute at Chicago.
Associate Professor at Johns Hopkins University
Scientists aim to extract simplicity from observations of the complex world. With data at hand, they look for trends but this process typically is more an art than a science. I will show how to think about this problem generically and present a new tool, the Sequencer (http://sequencer.org), which is able to automatically find trends in arbitrary datasets, without any training. I will then present some of its recent discoveries in astronomy and geology.
Brice Ménard joined the faculty at Johns Hopkins in 2010. He received his Ph. D. from both the Institut d’Astrophysique de Paris and the Max Planck Institute for Astrophysics in Germany. He was a postdoctoral member of the Institute for Advanced Study in Princeton and a senior research associate at the Canadian Institute for Theoretical Astrophysics in Toronto. His research combines astrophysics and statistics. His work has led to the detection of gravitational magnification by dark matter around galaxies, the discovery of tiny grains of dust in the intergalactic space, a new technique to estimate the redshift (or distance) of extragalactic objects and a new way to automatically find trends in complex datasets.
Ménard is a joint member of the Kavli Institute for Physics and Mathematics at Tokyo University. He received the Johns Hopkins President Frontier award (2019), the Packard fellowship for Science and Engineering (2014), the Sloan Research fellowship (2012), was named the 2012 Outstanding Young Scientist of Maryland, and was awarded the 2011 Henri Chrétien grant award by the American Astronomical Society.
at the Johns Hopkins University.
PI: Natalia Trayanova (Biomedical Engineering, WSE)Co-I: David Spragg (Cardiology, SOM), Nikhil Paliwal (Alliance for Cardiovascular Diagnostic and Treatment Innovation)
To prevent recurrent ablation procedures in atrial fibrillation (AF) patients, we propose a data-driven technology that will enable a priori prediction of the success of pulmonary vein isolation (PVI). We will use existing AF patient clinical data and artificial intelligence to train predictive models for the success of PVI using catheter ablation. The overall goal of this technology is to provide clinical guidance as to which AF patients would benefit from PVI, thus maximizing the benefit of PVI while minimizing the financial costs and procedural risks of unnecessary ablation procedures.
Nikhil is a postdoctoral fellow in the Alliance for Cardiovascular Diagnostic and Treatment Innovation (ADVANCE) at the Johns Hopkins University. He completed his PhD in Mechanical Engineering from the State University of New York at Buffalo (2019) and B. Tech in Bioengineering from the Indian Institute of Technology Kanpur, India (2011). His research interests include use of computational modeling and artificial intelligence to explore and predict clinical outcomes of cardiovascular diseases. His current work involves predictions of stroke risk and recurrence in patients with atrial fibrillation after catheter ablation, using electrophysiological and hemodynamic simulations along with machine learning.
Professor & Dunn Family Endowed Chair in Data Theory, Department of Mathematics, UCLA
Online Matrix Factorization (OMF) is a fundamental tool for dictionary learning problems, giving an approximate representation of complex data sets in terms of a reduced number of extracted features. Convergence guarantees for most of the OMF algorithms in the literature assume independence between data matrices, and the case of dependent data streams remains largely unexplored. In this talk, we present results showing that a non-convex generalization of the well-known OMF algorithm for i.i.d. data converges almost surely to the set of critical points of the expected loss function, even when the data matrices are functions of some underlying Markov chain satisfying a mild mixing condition. As the main application, by combining online non-negative matrix factorization and a recent MCMC algorithm for sampling motifs from networks, we propose a novel framework of Network Dictionary Learning that extracts `network dictionary patches’ from a given network in an online manner that encodes main features of the network. We demonstrate this technique on real-world data and discuss recent extensions and variations.
Deanna Needell earned her PhD from UC Davis before working as a postdoctoral fellow at Stanford University. She is currently a full professor of mathematics at UCLA. She has earned many awards including the IEEE Best Young Author award, the Hottest paper in Applied and Computational Harmonic Analysis award, the Alfred P. Sloan fellowship, an NSF CAREER and NSF BIGDATA award, and the prestigious IMA prize in Applied Mathematics. She has been a research professor fellow at several top research institutes including the Mathematical Sciences Research Institute and Simons Institute in Berkeley. She also serves as associate editor for IEEE Signal Processing Letters, Linear Algebra and its Applications, the SIAM Journal on Imaging Sciences, and Transactions in Mathematics and its Applications as well as on the organizing committee for SIAM sessions and the Association for Women in Mathematics.